Oral Presentations Group 1B
Tracks
Parallel Session 1
Tuesday, June 18, 2024 |
16:00 - 17:00 |
Lecture Theatre 1 |
Speaker
Dr Mark Pocklington
ST1
Nottingham University Hospitals NHS Trust
Groove Pancreatitis: A Histopathological Perspective from a Single Tertiary Care Centre
16:00 - 16:15Abstract
Background
Groove pancreatitis (GP) is a rare subtype of chronic segmental pancreatitis, characterised by fibrosis within the pancreaticoduodenal groove. This small potential space permits passage of the pancreatic ducts, duodenal papillae and small vessels, and is a site where numerous benign and neoplastic entities arise. The similitude of clinicoradiological features of GP with malignant diseases make the management of pancreaticoduodenal groove lesions complex, often resulting in surgical intervention. Histological examination of pancreaticoduodenal resection specimens remains the definitive method of diagnosis1,2.
Purpose
We present an insight into our experiences of GP, a challenging and often misdiagnosed pathological entity, specifically the histopathological features and associated benign and malignant diseases.
Methods
A retrospective review of cases with identified GP from pancreaticoduodenectomy specimens was undertaken from a single tertiary centre between 2011 and 2024. Associations with GP were evaluated, including coexisting primary malignancies, and features of concurrent benign pathology.
Results
Groove pancreatitis was identified in 18 patients following pancreaticoduodenectomy for presumed cancer (10 male, 8 female, median age 59 years, range 42-77 years). Eleven (61%) were diagnosed histologically with a malignancy, where six (55%) were pancreatic neuroendocrine tumours, and five (45%), pancreatic adenocarcinomas. Median tumour grading was pT3. In three (43%) of seven patients with benign diagnoses, GP was the only pathology. All 18 patients had histological evidence of concurrent benign pathology. Seven (39%) had chronic cholecystitis and five (28%), pancreatic atrophy. Brunner’s gland hyperplasia was observed three-times more frequently in patients without underlying malignancy.
Conclusions
Groove pancreatitis is a rare pathological entity with clinicoradiological semblances to pancreaticoduodenal malignancy. The histological diagnosis is essential for navigating ongoing care and features include medial duodenal wall thickening with cystic degeneration, fibro-inflammatory changes, and a varying incidence of Brunner’s gland hyperplasia, which may be observed more frequently in benign pathology associated with GP1.
Groove pancreatitis (GP) is a rare subtype of chronic segmental pancreatitis, characterised by fibrosis within the pancreaticoduodenal groove. This small potential space permits passage of the pancreatic ducts, duodenal papillae and small vessels, and is a site where numerous benign and neoplastic entities arise. The similitude of clinicoradiological features of GP with malignant diseases make the management of pancreaticoduodenal groove lesions complex, often resulting in surgical intervention. Histological examination of pancreaticoduodenal resection specimens remains the definitive method of diagnosis1,2.
Purpose
We present an insight into our experiences of GP, a challenging and often misdiagnosed pathological entity, specifically the histopathological features and associated benign and malignant diseases.
Methods
A retrospective review of cases with identified GP from pancreaticoduodenectomy specimens was undertaken from a single tertiary centre between 2011 and 2024. Associations with GP were evaluated, including coexisting primary malignancies, and features of concurrent benign pathology.
Results
Groove pancreatitis was identified in 18 patients following pancreaticoduodenectomy for presumed cancer (10 male, 8 female, median age 59 years, range 42-77 years). Eleven (61%) were diagnosed histologically with a malignancy, where six (55%) were pancreatic neuroendocrine tumours, and five (45%), pancreatic adenocarcinomas. Median tumour grading was pT3. In three (43%) of seven patients with benign diagnoses, GP was the only pathology. All 18 patients had histological evidence of concurrent benign pathology. Seven (39%) had chronic cholecystitis and five (28%), pancreatic atrophy. Brunner’s gland hyperplasia was observed three-times more frequently in patients without underlying malignancy.
Conclusions
Groove pancreatitis is a rare pathological entity with clinicoradiological semblances to pancreaticoduodenal malignancy. The histological diagnosis is essential for navigating ongoing care and features include medial duodenal wall thickening with cystic degeneration, fibro-inflammatory changes, and a varying incidence of Brunner’s gland hyperplasia, which may be observed more frequently in benign pathology associated with GP1.
Professor Elizabeth Soilleux
Professor Of Diagnostics And Biomarkers
University of Cambridge
Interpretable Machine Learning based detection of coeliac disease: the human way
16:15 - 16:30Abstract
Background: Coeliac disease (CD) diagnosis generally depends on histological examination of duodenal biopsies. However, the agreement between pathologist when diagnosing coeliac disease is estimated to be only 80%. This motivates the use of AI to improve the quality of diagnosis. However, most AI models are “black-box” methods – they do not justify or explain their outcome, hence hindering clinical adoption.
Purpose: We aim to improve the accuracy of coeliac disease diagnosis by developing an interpretable Machine Learning-based approach that imitates the approach taken by pathologists when diagnosing coeliac disease. Pathologists evaluate the level of crypt hyperplasia, villous blunting and intraepithelial lymphocytosis when diagnosing coeliac disease.
Methods: We present a semantic segmentation algorithm that can locate and classify villi, crypts, intraepithelial lymphocytes, as well as enterocytes in a Whole-Slide-Image of a duodenal biopsy. We use the segmentation masks to diagnose coeliac disease in an interpretable manner.
Results: Our model achieves a segmentation accuracy of 90% and manages to classify with an accuracy of 80%.
Conclusions: We highlight the potential for interpretable Machine Learning to be used to assist the pathologist and improve the accuracy of coeliac disease diagnosis.
Purpose: We aim to improve the accuracy of coeliac disease diagnosis by developing an interpretable Machine Learning-based approach that imitates the approach taken by pathologists when diagnosing coeliac disease. Pathologists evaluate the level of crypt hyperplasia, villous blunting and intraepithelial lymphocytosis when diagnosing coeliac disease.
Methods: We present a semantic segmentation algorithm that can locate and classify villi, crypts, intraepithelial lymphocytes, as well as enterocytes in a Whole-Slide-Image of a duodenal biopsy. We use the segmentation masks to diagnose coeliac disease in an interpretable manner.
Results: Our model achieves a segmentation accuracy of 90% and manages to classify with an accuracy of 80%.
Conclusions: We highlight the potential for interpretable Machine Learning to be used to assist the pathologist and improve the accuracy of coeliac disease diagnosis.
Professor Elizabeth Soilleux
Professor Of Diagnostics And Biomarkers
University of Cambridge
Clinical-grade detection of coeliac disease with computational pathology
16:30 - 16:45Abstract
Background: Coeliac disease diagnosis generally depends on histological examination of duodenal biopsies. However, the agreement between pathologist when diagnosing coeliac disease is estimated to be only 80%.
Purpose: We aim to improve the accuracy of coeliac disease diagnosis by developing a Machine Learning-based diagnostic approach.
Methods: We present a machine learning model that can detect the presence or absence of coeliac disease from a set of representative duodenal biopsies. We train our model on a diverse dataset with biopsies from four different hospitals using the Multiple-Instance-Learning paradigm in a supervised manner.
Results: Our model successfully diagnoses coeliac disease on an independent test set from a previously unseen source with an accuracy of over 96%, achieving both sensitivity and specificity above 95%, thereby showing the potential to outperform pathologists.
Conclusions: We highlight the potential for Machine Learning to be used to greatly improve the accuracy of coeliac disease diagnosis.
Purpose: We aim to improve the accuracy of coeliac disease diagnosis by developing a Machine Learning-based diagnostic approach.
Methods: We present a machine learning model that can detect the presence or absence of coeliac disease from a set of representative duodenal biopsies. We train our model on a diverse dataset with biopsies from four different hospitals using the Multiple-Instance-Learning paradigm in a supervised manner.
Results: Our model successfully diagnoses coeliac disease on an independent test set from a previously unseen source with an accuracy of over 96%, achieving both sensitivity and specificity above 95%, thereby showing the potential to outperform pathologists.
Conclusions: We highlight the potential for Machine Learning to be used to greatly improve the accuracy of coeliac disease diagnosis.
Miss Zexi Li
Other
University Of Oxford
Comparison of the Performance of the Whole Slide Image Quality Assessment Tool PathProfiler across Three Tissue Type Cohorts
16:45 - 17:00Abstract
Background
The advancement of artificial intelligence algorithms in histopathology has led to the establishment of quality assessment (QA) tools for whole slide image (WSI) quality. Image quality for accurate assessment is key for both research and clinical diagnosis as it may affect downstream treatment or AI model performance. PathProfiler is a previously developed QA tool for use in prostate biopsy cohorts, predicting the overall useability.
Purpose
We previously evaluated PathProfiler performance in a time-limited prospective study in bladder transurethral resection and kidney resection cases but these were limited in number in that assessment. In this study, PathProfiler was examined in larger cohorts, aiming to assess its generalisability in non-prostate histopathological images.
Methods
The latest version of PathProfiler was tested on three retrospective cohorts where 1052 slides had been scanned in the diagnostic histopathology laboratory comprising biopsies from prostate (331 slides), kidney (363 slides), and bladder samples (358 slides). The slide-level usability scores (0-1 with threshold = 0.5) with out-of-focus and staining quality scores (1-10 with threshold = 9) were generated and compared across the three cohorts.
Results
PathProfiler demonstrated good accuracy in predicting usability scores for prostate (83%) and kidney (80%) WSIs. However, it displayed a higher false-negative rate (28%) and lower accuracy (71%) for bladder cohort, particularly struggling with distinguishing between out-of-focus areas and black ink artefacts. The useability threshold varies among cohorts and requires further tests to be calculated.
Conclusions
Our findings suggest that the prostate biopsy pre-trained PathProfiler has the potential to be applied to urological QA tests in kidney and bladder specimens, giving more insight into PathProfiler in these specimens. It has the potential to yield high performance in predicting usability beyond prostate samples despite not being trained previously on these tissue types. Further training may be needed to optimize its performance across diverse histopathological images.
The advancement of artificial intelligence algorithms in histopathology has led to the establishment of quality assessment (QA) tools for whole slide image (WSI) quality. Image quality for accurate assessment is key for both research and clinical diagnosis as it may affect downstream treatment or AI model performance. PathProfiler is a previously developed QA tool for use in prostate biopsy cohorts, predicting the overall useability.
Purpose
We previously evaluated PathProfiler performance in a time-limited prospective study in bladder transurethral resection and kidney resection cases but these were limited in number in that assessment. In this study, PathProfiler was examined in larger cohorts, aiming to assess its generalisability in non-prostate histopathological images.
Methods
The latest version of PathProfiler was tested on three retrospective cohorts where 1052 slides had been scanned in the diagnostic histopathology laboratory comprising biopsies from prostate (331 slides), kidney (363 slides), and bladder samples (358 slides). The slide-level usability scores (0-1 with threshold = 0.5) with out-of-focus and staining quality scores (1-10 with threshold = 9) were generated and compared across the three cohorts.
Results
PathProfiler demonstrated good accuracy in predicting usability scores for prostate (83%) and kidney (80%) WSIs. However, it displayed a higher false-negative rate (28%) and lower accuracy (71%) for bladder cohort, particularly struggling with distinguishing between out-of-focus areas and black ink artefacts. The useability threshold varies among cohorts and requires further tests to be calculated.
Conclusions
Our findings suggest that the prostate biopsy pre-trained PathProfiler has the potential to be applied to urological QA tests in kidney and bladder specimens, giving more insight into PathProfiler in these specimens. It has the potential to yield high performance in predicting usability beyond prostate samples despite not being trained previously on these tissue types. Further training may be needed to optimize its performance across diverse histopathological images.
Chair
Mark Arends
President & Professor Of Pathology
University Of Edinburgh
Marnix Jansen
UCL
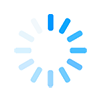