Plenary Session 1
Tracks
Parallel Session 1
Parallel Session 2
Parallel Session 3
Parallel Session 4
Wednesday, June 19, 2024 |
14:00 - 15:30 |
Lecture Theatre 1 |
Speaker
Miss Jane Ganeshalingam
MBPhD student at UCL Cancer Institute
UCL
Modelling ETV6::RUNX1+ B-cell ALL: Secondary loss of the wild-type ETV6 allele releases the B-cell developmental block imposed by first-hit ETV6::RUNX1
14:00 - 14:15Abstract
Introduction:
ETV6::RUNX1 is the most frequent genetic aberration in childhood B-cell acute lymphoblastic leukemia (B-ALL), the most common pediatric cancer, and acts as a ‘first-hit’; arising in utero but resulting in a clinically silent pre-leukemia. Additional post-natal mutations are necessary for leukemic transformation, but how they collaborate with the initiating event has not been fully characterized. iPSCs recapitulate fetal lymphopoiesis, affording an opportunity to model leukemogenesis in developmentally relevant cell types. In this system, engineered ETV6::RUNX1 corrupts B-myeloid (CD34+CD19-IL7R+) progenitors and blocks B-cell differentiation¹.
Methods:
Loss of the wild-type ETV6 allele is the most frequent second-hit in ETV6::RUNX1+ B-ALL. Using CRISPR/Cas9-mediated mutagenesis, ETV6::RUNX1/ETV6-/- lines were established; subsequent cre-mediated reversion of ETV6::RUNX1 derived ETV6-/- clones. In vitro B-lymphopoiesis was assessed by flow-cytometry and immunophenotypically-defined B-myeloid, proB and preB compartments were sorted for RNA-sequencing, then subjected to differential gene expression and pathway analyses.
Results:
Secondary loss of the wild-type ETV6 allele resulted in expansion of the B-myeloid progenitor and downstream proB and preB populations. Transcriptomic analysis revealed upregulation of genes enriched in signatures of cell cycle, B-cells and inflammatory pathways following additional mutation of ETV6 in the B-myeloid progenitor compartment. Reversion of ETV6::RUNX1 revealed that B-myeloid progenitor expansion and inflammatory signature upregulation are dependent on a first-/second-hit interaction. Additionally, a significant upregulation of RAG1/RAG2 gene expression is observed in ETV6::RUNX1/ETV6-/- preB cells.
Conclusion:
Our results suggest that secondary loss of ETV6 promotes leukemogenesis by: i) upregulating cell cycle and B-cell associated signatures, amplifying the B-myeloid progenitor compartment and releasing the ETV6::RUNX1 block on B-cell differentiation; ii) activating or sensitizing to inflammatory signaling which may additionally iii) collaborate with RAG upregulation in preB cells to promote subsequent genomic rearrangements. Work is ongoing to characterize the role of other second-hit mutations (PAX5+/- and CDKN2A-/-), individually and in combination, in childhood ETV6::RUNX1+ B-ALL.
ETV6::RUNX1 is the most frequent genetic aberration in childhood B-cell acute lymphoblastic leukemia (B-ALL), the most common pediatric cancer, and acts as a ‘first-hit’; arising in utero but resulting in a clinically silent pre-leukemia. Additional post-natal mutations are necessary for leukemic transformation, but how they collaborate with the initiating event has not been fully characterized. iPSCs recapitulate fetal lymphopoiesis, affording an opportunity to model leukemogenesis in developmentally relevant cell types. In this system, engineered ETV6::RUNX1 corrupts B-myeloid (CD34+CD19-IL7R+) progenitors and blocks B-cell differentiation¹.
Methods:
Loss of the wild-type ETV6 allele is the most frequent second-hit in ETV6::RUNX1+ B-ALL. Using CRISPR/Cas9-mediated mutagenesis, ETV6::RUNX1/ETV6-/- lines were established; subsequent cre-mediated reversion of ETV6::RUNX1 derived ETV6-/- clones. In vitro B-lymphopoiesis was assessed by flow-cytometry and immunophenotypically-defined B-myeloid, proB and preB compartments were sorted for RNA-sequencing, then subjected to differential gene expression and pathway analyses.
Results:
Secondary loss of the wild-type ETV6 allele resulted in expansion of the B-myeloid progenitor and downstream proB and preB populations. Transcriptomic analysis revealed upregulation of genes enriched in signatures of cell cycle, B-cells and inflammatory pathways following additional mutation of ETV6 in the B-myeloid progenitor compartment. Reversion of ETV6::RUNX1 revealed that B-myeloid progenitor expansion and inflammatory signature upregulation are dependent on a first-/second-hit interaction. Additionally, a significant upregulation of RAG1/RAG2 gene expression is observed in ETV6::RUNX1/ETV6-/- preB cells.
Conclusion:
Our results suggest that secondary loss of ETV6 promotes leukemogenesis by: i) upregulating cell cycle and B-cell associated signatures, amplifying the B-myeloid progenitor compartment and releasing the ETV6::RUNX1 block on B-cell differentiation; ii) activating or sensitizing to inflammatory signaling which may additionally iii) collaborate with RAG upregulation in preB cells to promote subsequent genomic rearrangements. Work is ongoing to characterize the role of other second-hit mutations (PAX5+/- and CDKN2A-/-), individually and in combination, in childhood ETV6::RUNX1+ B-ALL.
Dr Alistair Curd
Research Fellow
University Of Leeds
Super-resolution fluorescence microscopy and AI for renal diagnosis: a replacement for electron microscopy?
14:15 - 14:30Abstract
Super-resolution fluorescence microscopy (SRFM) can provide data on the spatial distributions of specific proteins at a level of detail well beyond the capability of standard immunofluorescence or confocal microscopy (~20 nm). Compared with electron microscopy (EM), it is also easy to fit into standard FFPE tissue processing protocols [1, 2], faster overall as an assay and highly specific for molecules of interest. It may also conveniently image more regions of interest in a tissue section than EM, potentially allowing greater sensitivity to pathological changes.
Renal disease is the most common use case for EM as a diagnostic assay. In particular, focal segmental glomerulosclerosis (FSGS) and minimal change disease (MCD) currently require EM to inspect the morphology of podocytic structures on the glomerular capillary basement membrane.
We have acquired SRFM data on nephrin, which localises to the filtration slits between podocyte foot processes, and collagen, part of the basement membrane. We have obtained more than 300 fields of view (FOVs) in total for each protein, from FFPE tissue sections from several MCD, FSGS (both diagnosed according the current gold standard) and normal control cases (sections from donors with no known renal disease).
We have trained an AI algorithm to classify the FOVs as MCD, FSGS or Normal, using the gold standard diagnoses as ground truth. We partition the FOVs into multiple tiles and apply an attention-based multiple-instance learning pipeline, to make use of and identify salient areas in a FOV [3, 4]. We have obtained a mean AUROC > 0.90 in 5-fold cross-validation for predicting all three conditions.
SRFM has the potential to provide a wealth of new information relevant to medical decision-making. We also anticipate that it will save time and cost, and in some cases improve sensitivity, where EM is currently used.
S.B. was supported by a PathSoc grant.
Renal disease is the most common use case for EM as a diagnostic assay. In particular, focal segmental glomerulosclerosis (FSGS) and minimal change disease (MCD) currently require EM to inspect the morphology of podocytic structures on the glomerular capillary basement membrane.
We have acquired SRFM data on nephrin, which localises to the filtration slits between podocyte foot processes, and collagen, part of the basement membrane. We have obtained more than 300 fields of view (FOVs) in total for each protein, from FFPE tissue sections from several MCD, FSGS (both diagnosed according the current gold standard) and normal control cases (sections from donors with no known renal disease).
We have trained an AI algorithm to classify the FOVs as MCD, FSGS or Normal, using the gold standard diagnoses as ground truth. We partition the FOVs into multiple tiles and apply an attention-based multiple-instance learning pipeline, to make use of and identify salient areas in a FOV [3, 4]. We have obtained a mean AUROC > 0.90 in 5-fold cross-validation for predicting all three conditions.
SRFM has the potential to provide a wealth of new information relevant to medical decision-making. We also anticipate that it will save time and cost, and in some cases improve sensitivity, where EM is currently used.
S.B. was supported by a PathSoc grant.
Dr Kastytis Sidlauskas
Registrar
Barts Cancer Institute
Identifying Morphological Biomarkers for Progression of Ductal Carcinoma in Situ of the Breast Through Computational Analysis
14:30 - 14:45Abstract
Background
Ductal Carcinoma in situ (DCIS) is a pre-invasive breast cancer that may or may not progress to invasive cancer. Despite this uncertainty, all women diagnosed with DCIS undergo surgical excision and additional therapy. However, there are no reliable markers to differentiate between DCIS lesions that will progress or recur and those that will not.
Purpose
This study aims to distinguish morphological features across various DCIS nuclear types, to lay the groundwork for prognostic models.
Methods
We utilised HoVer-Net, fine-tuned with 50,000 manually annotated nuclei, for nuclear segmentation/classification in whole slide images. Additionally, the DeepLab model with a ResNet backbone facilitated 9-class region segmentation, distinguishing benign ducts, DCIS, fibrous stroma, among others. Using selected regions from 320 cases of the UK/ANZ DCIS trial, we assessed nuclear morphology and applied logistic regression and random forest models to identify prognostic features for DCIS progression.
Results
The DeepLab model, enhanced with a ResNet backbone, achieved a Dice score of 0.87 in the validation set, while the fine-tuned HoVer-Net exhibited an F1 score of 0.81 for nuclear classification. Our analysis discerned 17 morphological distinctions with statistical significance (p<0.05) between progressors and non-progressors, particularly in cellular orientation, eccentricity and solidity of lymphocytes and nuclear radii, perimeter and orientation within neoplastic nuclei. The logistic regression and random forest findings were consistent. The convergence of these analytical models highlighted consistent predictive features, thereby refining our predictive capacity for DCIS progression and recurrence.
Conclusions
Through the development of a 9-class region segmentation and the refinement of HoVer-Net for nuclear segmentation and classification, we have identified significant morphological variations that serve as potential prognostic indicators for the risk of DCIS progression. The integration of these features into an interpretable computational model, subject to external validation, represents the next phase of research, aimed at enhancing the prediction of DCIS progression.
Ductal Carcinoma in situ (DCIS) is a pre-invasive breast cancer that may or may not progress to invasive cancer. Despite this uncertainty, all women diagnosed with DCIS undergo surgical excision and additional therapy. However, there are no reliable markers to differentiate between DCIS lesions that will progress or recur and those that will not.
Purpose
This study aims to distinguish morphological features across various DCIS nuclear types, to lay the groundwork for prognostic models.
Methods
We utilised HoVer-Net, fine-tuned with 50,000 manually annotated nuclei, for nuclear segmentation/classification in whole slide images. Additionally, the DeepLab model with a ResNet backbone facilitated 9-class region segmentation, distinguishing benign ducts, DCIS, fibrous stroma, among others. Using selected regions from 320 cases of the UK/ANZ DCIS trial, we assessed nuclear morphology and applied logistic regression and random forest models to identify prognostic features for DCIS progression.
Results
The DeepLab model, enhanced with a ResNet backbone, achieved a Dice score of 0.87 in the validation set, while the fine-tuned HoVer-Net exhibited an F1 score of 0.81 for nuclear classification. Our analysis discerned 17 morphological distinctions with statistical significance (p<0.05) between progressors and non-progressors, particularly in cellular orientation, eccentricity and solidity of lymphocytes and nuclear radii, perimeter and orientation within neoplastic nuclei. The logistic regression and random forest findings were consistent. The convergence of these analytical models highlighted consistent predictive features, thereby refining our predictive capacity for DCIS progression and recurrence.
Conclusions
Through the development of a 9-class region segmentation and the refinement of HoVer-Net for nuclear segmentation and classification, we have identified significant morphological variations that serve as potential prognostic indicators for the risk of DCIS progression. The integration of these features into an interpretable computational model, subject to external validation, represents the next phase of research, aimed at enhancing the prediction of DCIS progression.
Dr Alexandra McLaren
ST3
NHS Greater Glasgow & Clyde
A Self-Supervised AI Learning Approach Extracts Morphological Meaning From H&E Images of Colorectal Cancer
14:45 - 15:00Abstract
Background: Diagnosis and treatment of colorectal cancer (CRC) is dependent upon microscopic assessment of information contained within tissue by pathologists. This is time and resource demanding and prone to inter- and intra-observer variability. Recent development in self-supervised learning approaches provide new opportunities to better understand the morphological characteristics of cancer on histological slides¹. We have applied a self-supervised learning artificial intelligence (AI) approach called Histomorphological Phenotype Learning (HPL) to whole slide images (WSIs) of CRC.
Purpose: To apply the self-supervised learning AI approach HPL to CRC in order to build a library of morphological clusters that represent regions of WSIs and assess their predictive potential.
Methods: We have trained a self-supervised learning Barlow Twins method on 2772 H&E WSIs of colorectal adenocarcinoma from the TranSCOT cohort. The WSIs were split into tiles that are 224x224 pixels at 10x magnification. The model was trained on a subset of 500,000 tiles and morphological features from these were extracted. We utilised Leiden community detection to identify histomorphological phenotype clusters (HPCs) in a features space defined by the learned representations, free from any clinical or pathological labels. A Cox proportional hazards model was applied over patient vector representations, defining each patient as a composition of HPCs. Tiles within each HPC have been examined by pathologists and the morphological features documented.
Results: Resulting HPCs display distinctive histomorphological patterns that highlight tissue type and architecture. HPCs achieve a concordance index range of 0.59 to 0.66. HPCs associated with poorer outcome are stroma rich and display poorly differentiated tumour. HPCs associated with better outcome display high immune cell infiltration.
Conclusions: Self-supervised learning AI approaches have the ability to predict outcome and provide a library of histomorphological features within tumour and tumour microenvironment.
This research has been supported by the Jean Shanks & Pathological Society Pre-Doctoral Research Bursary.
Purpose: To apply the self-supervised learning AI approach HPL to CRC in order to build a library of morphological clusters that represent regions of WSIs and assess their predictive potential.
Methods: We have trained a self-supervised learning Barlow Twins method on 2772 H&E WSIs of colorectal adenocarcinoma from the TranSCOT cohort. The WSIs were split into tiles that are 224x224 pixels at 10x magnification. The model was trained on a subset of 500,000 tiles and morphological features from these were extracted. We utilised Leiden community detection to identify histomorphological phenotype clusters (HPCs) in a features space defined by the learned representations, free from any clinical or pathological labels. A Cox proportional hazards model was applied over patient vector representations, defining each patient as a composition of HPCs. Tiles within each HPC have been examined by pathologists and the morphological features documented.
Results: Resulting HPCs display distinctive histomorphological patterns that highlight tissue type and architecture. HPCs achieve a concordance index range of 0.59 to 0.66. HPCs associated with poorer outcome are stroma rich and display poorly differentiated tumour. HPCs associated with better outcome display high immune cell infiltration.
Conclusions: Self-supervised learning AI approaches have the ability to predict outcome and provide a library of histomorphological features within tumour and tumour microenvironment.
This research has been supported by the Jean Shanks & Pathological Society Pre-Doctoral Research Bursary.
Chair
Mohammad Ilyas
Meetings Secretary
University Of Nottingham
Louise Jones
Professor Of Breast Pathology
Barts Cancer Institute, Queen Mary University Of London, London
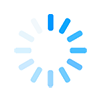